ROC’s SDK v2.2 is now the single-highest performing vendor of all U.S. FR algorithm providers in the latest National Institute of Standards and Technology (NIST) Face Recognition Vendor Test (FRVT). Specifically, v2.2 outranks all others with both top tier accuracy and efficiency in both the 1:1 and 1:N series. At 2-5x faster and 10-20x more efficient, v2.2 packs a punch of impressive computer vision developments: 5x improvement in liveness fraud detection, expanded PersonID searching and brand new LPR functionality. These performance improvements will directly enhance performance of ROC Watch and all ROC applications. Contact us to ensure you have up to date versions of our top-ranked SDK.
True to our company culture, ROC continues to deliver the most efficient, accurate, and trusted computer vision algorithms available on the market today. I’m incredibly proud of the work we have done in a few short months since releasing our next generation AI/ML-powered version 2.0. In that release, we included some exciting new computer vision capabilities including Liveness fraud prevention, Vehicle and Object Detection and License Plate Recognition (LPR) based on Optical Character Recognition (OCR). Read more about v2.0 in our previous blog.
With the v2.2 release, our AI/ML developers have made impressive gains that deliver powerful improvements to Liveness fraud prevention, LPR, and Person ID in addition to ground-breaking accuracy performance for Face Recognition – all in a single, efficient SDK packet.
Face Recognition Improvements
While accuracy metrics are the primary currency of FR vendors, integrators of FR systems know that real-world performance of these algorithms requires that they are deployable in real-world settings, such as high throughput video processing, large-scale enterprise search systems, or on-edge mobile use-cases. For this reason, ROC continues to prioritize speed and efficiency in parallel to our continued drive for top accuracy performance. And with v2.2, we have continued to establish ourselves as the industry leader.
Just as smart phones require chips that are small, efficient, and fast – FR applications require algorithms that are efficient, fast, and accurate. With ROC SDK v2.2, you don’t have to choose between accuracy and efficiency.
As shown in Figure 1 below from the 07/28/2022 NIST FRVT Ongoing report, Rank One is, by a wide margin, able to deliver the best combination of accuracy and efficiency when measuring the mean FRVT rankings in all eight accuracy benchmarks (Tables 18 to 27: Visa MC, Visa, Mugshot, Mugshot 12+Yrs, VisaBorder, Border 10-6, Border 10-5, Wild) and all four efficiency benchmarks (Tables 8 to 17: template generation speed, template size, binary size, and comparison speed.)
Figure 1: Mean Accuracy and Efficiency Ranking across 28 Commercial FR Vendors
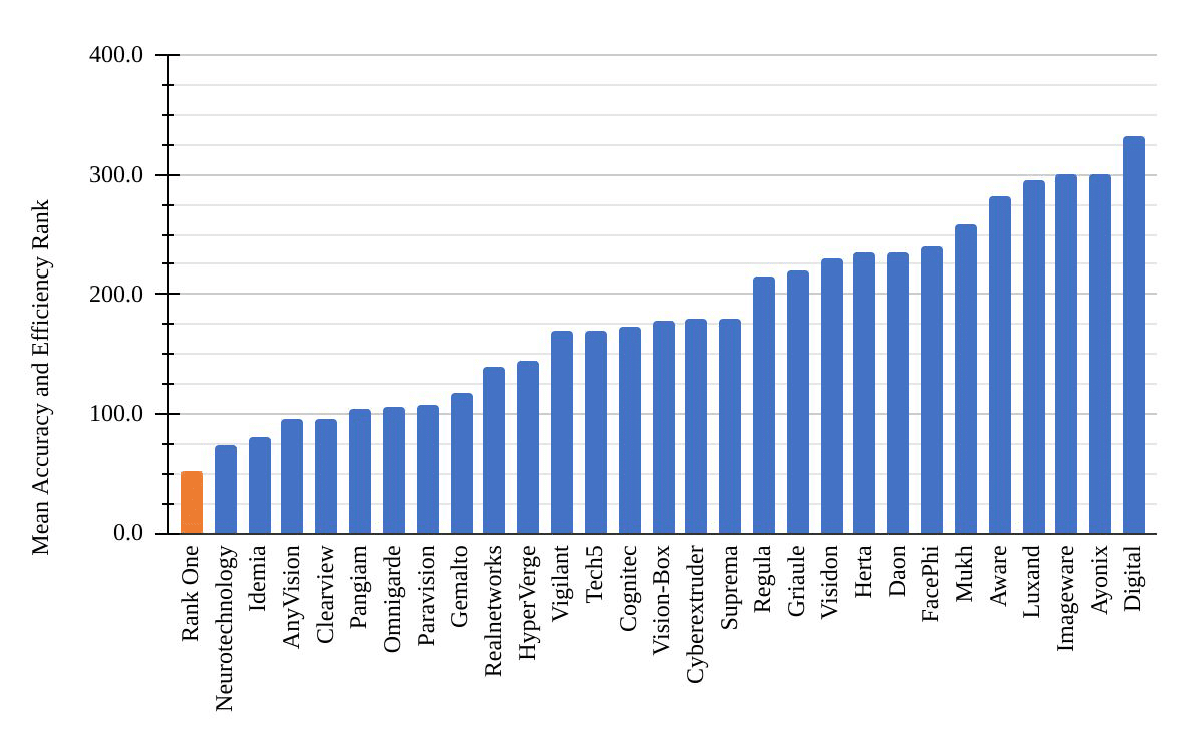
When focusing specifically on accuracy metrics, v2.2 continues ROC’s impressive climb in rankings. For example, as shown in Figure 2 below, ROC SDKv2.2 is ranked as the #1 most accurate U.S. vendor on Border crossing data (1E-5). v2.2 also ranked #6 globally of 440 algorithms total with all of the top 5 developed in China, Russia, and Korea.
Figure 2: Error Rates for on Border Data (1E-5) for U.S. Vendors
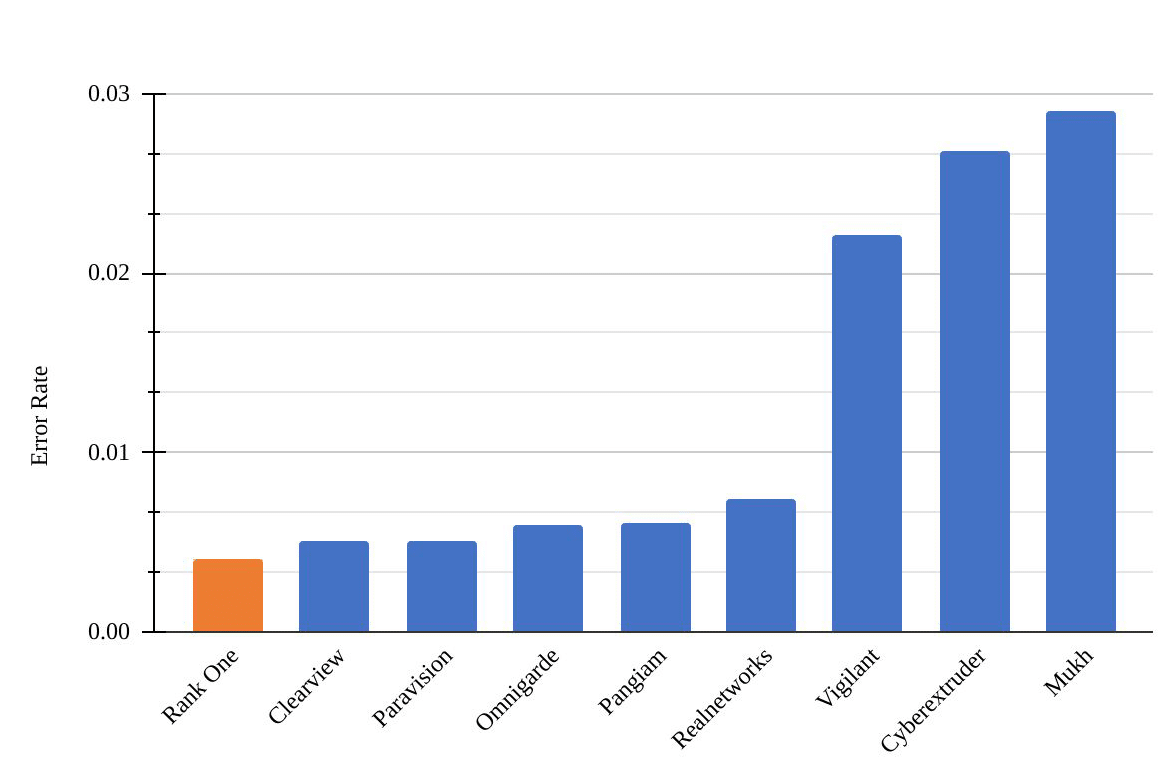
And more broadly, ROC v2.2 achieved better than 99.5% genuine match rate on all Border, Visa, and Mugshot datasets. Figure 3 demonstrates this as well as the dramatic 1.4x improvements that ROC has made in just the last six months.
Figure 3: ROC SDK v2.2 Error rate improvements over v2.0
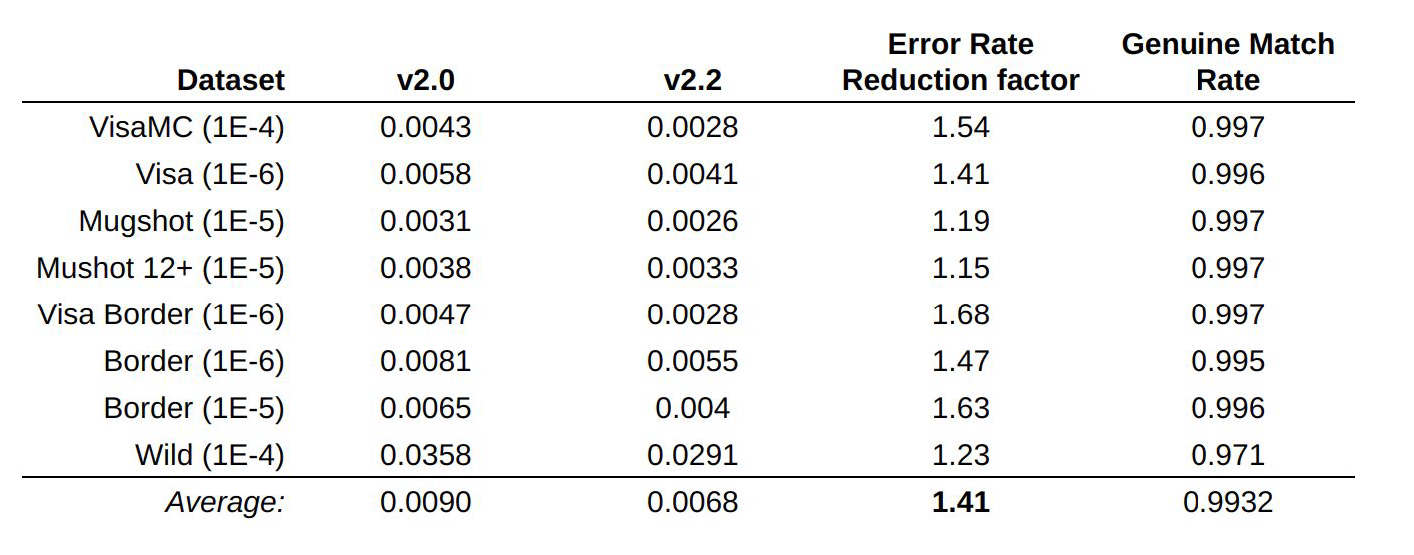
Liveness Fraud Prevention
As defined by the Presentation Attack Detection (PAD) standards outlined in ISO/IEC 30107-3, Liveness detection seeks to combat the following attempts to make a fraudulent FR match:
- 2D static attacks: high-definition face pictures on flat paper or simple flat paper masks with holes are presented to the FR matcher
- 2D dynamic hacks: multiple images (2D photos or 3D avatars) are played in sequence on a 2D screen
- 3D static attacks: impersonators use 3D prints, wax heads, or sculptures
- 3D dynamic attacks – impersonators use sophisticated masks to imitate liveness.
v2.2 achieved massive reductions in PAD error rates. Bona Fide Presentation Classification Error Rate (BPCER) reduced by 7x when operating at our “High Security” threshold and 5x when operating at our “Low Security” threshold. Attack Presentation Classification Error Rate (APCER) held constant at these operating thresholds.
New PersonID Functionality
PersonID refers to the capability to recognize persons through morphology (shapes and colors). v2.2 adds the ability to use clothing color semantics to query a video feed for persons wearing such clothing. For example, using v2.2 an analyst query can be performed to “Find all images that include a person with a yellow shirt and gray pants.” Additionally, the ability to compare the visual similarity of two persons using computer vision representations is still supported in the ROC SDK. Together, this PersonID functionality supports both real-time monitoring and forensic investigation use-cases.
Expanded License Plate Recognition
Building on the v2.0 debut of LPR, v2.2 tackles specific challenges associated with recognizing the many different license plate formats and layouts issued by states, municipalities, and agencies. This release delivers major improvements in the ability to detect the U.S. state of origin for license plates and to accurately recognize license plate digits using Optical Character Recognition (OCR). To achieve these results, ROC developed tailored training data for its AI/ML algorithms to improve performance in operationally relevant conditions.
About Rank One
Founded in 2015, ROC is an employee-owned company with headquarters in Denver, CO and offices in Morgantown, WV that develops its software entirely in-house and in the U.S.A. ROC delivers top performing face recognition and computer vision algorithms with a no-nonsense business approach and deep commitment to best practices in software engineering and pattern recognition algorithm design. We have initiated – and continue to lead – the charge to develop responsible AI by establishing the FR industry’s first code of ethics that governs our development and deployment of AI/ML algorithms and software in both commercial and government applications.